I’ve been working for some years now in distributed systems and event-driven architectures, from the misunderstood SOA (or its refurbished version known as Microservices) to Event Sourcing.
Some of the concepts presented in these systems related to events like immutability, perpetuity and versioning are valid as well for stream processing. Stream processing along with batch processing is sometimes referred as Big Data.
Big Data
When we think about Big Data what it first comes to our mind is Hadoop for batch processing. Although Hadoop has a big capacity to process indecent amounts of data, it also comes with a high latency response.
Although this latency won’t be a problem for a lot of use cases, it may be a problem when we need to get real (or near-real) time feedback.
That’s where the Lambda Architecture (by Nathan Marz) comes in by describing how to design a system where most of our data is processed by the batch layer but, while this process is running, we are able to process the streams coming into our system:
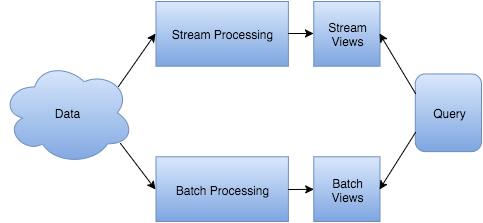
Where we can say that:
Current View = Query(Batch View) + Query(Stream View)
Batch Layer
The batch processing layer computes arbitrary sets of data using the entire historical data. The obvious example of batch processing is Hadoop, or to be more precise, the distributed file system HDFS and a processing tool like MapReduce, Pig…
The result of this process will be stored in a database that should support batch writes (ElephantDB, HBase) but no random writes. That makes the database architecture extremely simple by removing features like online compactation or concurrency.
Stream Layer
The stream processing layer computes data one by one giving immediate feedback. Depending on the number of events or the throughput needed we may use different technologies: Spark Streaming (although it’s micro-batch the latency may be sufficient for many use cases), Storm, Samza, Flink.
The result of this process will be stored in a database that should support random writes, one option may be Cassandra.
In following posts I will present concrete examples with docker images using some technologies that I’ve used like: Kafka, Storm, Cassandra and Druid.